JOWO 2022: Keynote Speakers
We proudly present our confirmed keynote speakers.
Peter Gärdenfors
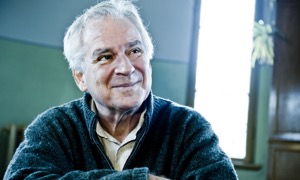
Title
Conceptual spaces, event structure and cognitive ontology
Abstract
I first give a brief introduction to the theory of conceptual spaces. Then I present a model of events where the main constituents are agents, patients, force vectors and results vectors. I show how the constituents can be described in terms of conceptual spaces. I also explain how the event model is related to causal thinking. More importantly, the model forms the basis for a cognitive ontology that is required for developing a semantics for natural language in artificial systems.
Bio
Gärdenfors is a Senior Professor of Cognitive Science at Lund University. His main research areas include concept formation, cognitive semantics and the evolution of cognition. He is a member of the Royal Swedish Academy of Sciences; the Royal Swedish Academy of Letters, History and Antiquities; Academia Europaea; Leopoldina Deutsche Akademie für Naturforscher; and the Royal Swedish Academy of Engineering Sciences. Additionally, he is a member of the Prize Committee for the Prize in Economic Sciences in Memory of Alfred Nobel 2011-2017 and a fellow of the Cognitive Science Society.
He has published close to 300 articles and scientific contributions, but his main books are: Knowledge in Flux: Modeling the Dynamics of Epistemic States (MIT Press 1988), Conceptual Spaces: The Geometry of Thought (MIT Press 2000), and Geometry of Meaning: Semantics Based on Conceptual Spaces (MIT Press 2014).
In addition to his scientific pursuits, Gärdenfors has a black belt in Judo, is an amateur botanist and a true vagabond that travels extensively all over the world.
Sebastian Rudolph – Invited by the FMKD workshop
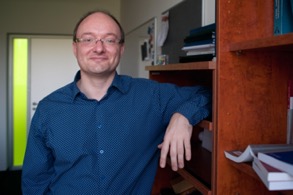
Title
The Matrix Has You – Toward Compositional Conceptual Spaces
Abstract
It has often been argued that certain aspects of meaning can or should be represented geometrically, e.g. by means of conceptual spaces. In fact, vector space models already have quite some tradition in language technologies and recently regained interest under the term embeddings in the context of deep learning. The principle of compositionality, going back to Frege, states that the meaning of a complex language construct is a function of its constituents' meanings. A significant body of work in computational linguistics investigates if and how the principle of compositionality can be applied to geometrical models. A decade ago, compositional matrix space models (CMSMs) were proposed as a unified framework capable of capturing many of the common vector-based composition functions. During the past ten years, more results have been obtained regarding the learnability of CMSMs and their feasibility for practical NLP tasks. This talk gives an overview of theoretical and practical aspects of CMSMs.
Bio
Sebastian Rudolph is a full professor of Computational Logic at TU Dresden. His research interests focus on the mathematical and computational foundations of formal approaches to Knowledge Representation and Reasoning, including their practical applications in diverse areas, also encompassing methodological questions of ontological modeling and interactive knowledge acquisition.
As part of the W3C working group, Sebastian contributed to the standardization of the
second edition of the Web Ontology Language (OWL 2) and co-authored popular textbooks on the foundations of semantic web technologies. He also co-authored more than 150 conference and journal publications in the fields of Artificial Intelligence, Database Theory, Computational Logic, and Natural Language Processing.
In 2017, he received an ERC Consolidator Grant for investigating general principles of decidability in logic-based knowledge representation.
Janna Hastings
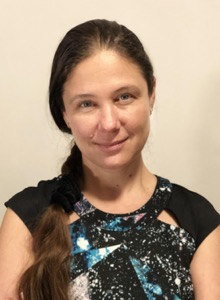
Abstract
The last decade has seen rapid advances in use of artificial intelligence technologies, mostly based on 'deep learning', to solve real-world problems. However, these systems suffer from characteristic weaknesses, for example, they cannot directly explain their predictions, they struggle to learn meaningful generalisations and apply them appropriately in scenarios far removed from their training datasets, and they frequently amplify biases they have learned from data. Ontologies, as symbolic representational artifacts, are formalized in logical languages that allow verifiable behaviour and inferences. In this presentation I will discuss the essential roles of modern ontologies in improving deep learning by enabling hierarchical generalisations and the assignment of meaningful constraints. I will illustrate the discussion with practical examples drawn from chemistry, medicine and behavioural science.
Bio
Janna Hastings holds a PhD in Biological Sciences from the University of Cambridge, an MSc in Computer Science from the University of South Africa, and an MA in Social and Political Philosophy from the UK's Open University. She is currently Assistant Professor of Medical Knowledge and Decision Support at the Medical Faculty of the University of Zurich and the School of Medicine at the University of St. Gallen. Her research in applied ontologies spans more than a decade encompassing both their development and their use in intelligent systems. The current focus of her research is on the use of knowledge-aware systems to accelerate health evidence synthesis and translation in practice to support clinicians and patients in making informed decisions, as well as to better understand the impact of automated information systems and their semantic commitments on patient and clinician decision-making processes.
See JOWO 2022 workshops and tutorials.
Contact:
JOWO 2022 Chairs: jsteering.JOWO2022@gmail.com
JOWO Steering Committee: jowo.steering@gmail.com